Use the Power of Historical Data
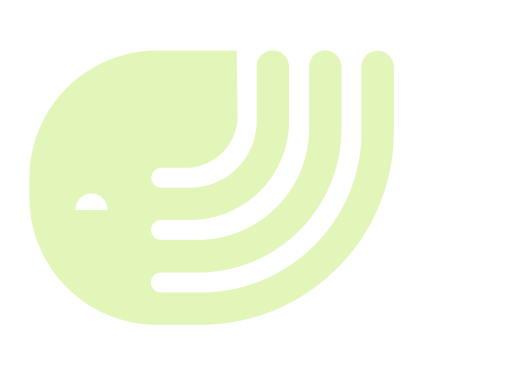
Mar 15, 2024
Mads Brodthagen & Anders Valentin Vogt
Introduction
In the complex world of commodity trading, the saying "history repeats itself" serves not merely as a philosophical thinking but as a foundational principle that supports investment and trading strategies. This is particularly relevant in the context of market cycles, which are known for their tendency to exhibit patterns and behaviours that recur over time. By closely examining past market trends and cycles, traders and investors can accumulate insights into potential future movements, allowing them to make more informed decisions. This article dives into the value of historical data analysis for forecasting market trends.
What makes Historical Data so powerful?
Historical data proves to be an indispensable tool for those who want to stay ahead of the dynamic movements of commodity- and freight markets. The strategic use of historical data in commodity trading is crucial for portfolio diversification, risk management, and the timing of market entries and exits. It enables traders and investors to utilize past market responses to various stimuli—such as economic cycles, policy changes, and supply disruptions— allowing them to effectively understand the correlation to market variables such as commodity futures, cash prices, and freight markets and thus it provides a solid foundation to analyse these market dynamics.
The impact of geopolitical events on commodity prices is a key example of historical data's relevance. As an example, oil markets are significantly affected by geopolitical tensions in the Middle East, where production disruptions have historically led to sharp increases in global oil prices. By analysing episodes like the oil shocks of the 1970s or more recent conflicts in oil-producing regions, analysts can build models to better anticipate the effects of similar future incidents on commodity markets (Carter, Rausser & Smith, 2011). Furthermore, the analysis of historical freight rates serves as a critical component of the dry bulk commodity trading equation, providing insights into broader economic and logistical trends. These insights reflect the multifaceted influence of numerous factors on the markets and underscore the importance of historical data in uncovering supply-demand correlations, price elasticity, political impacts, and market sentiment over time (Barkoulas, Hu & Santos, 2008).
The Role of Historical Data in Developing Predictive Models
Predictive Models live
Predictive modelling in commodity trading exceeds mere speculation, anchoring itself in the systematic interpretation of correlations of historical data. The integration of historical data allows statistical models, machine learning algorithms, and other analytical tools to uncover trends that might otherwise not be immediately apparent. Historical variables might include lagged prices, historical volatility indices, and even sentiment analysis derived from news archives. The latter approach enables the formulation of forecasts hypothesises that predict market behaviour with a higher degree of accuracy than traditional methods, as proved by Pai, Hong, and Lin (2018) in the realm of stock price forecasting.
Traditional statistical modelling techniques, such as Linear Regression and Autoregressive Integrated Moving Average (ARIMA) models, have long been central in forecasting commodity prices. Linear Regression offers a straightforward approach by establishing linear relationships between variables, while ARIMA models capture the temporal dependencies and seasonality inherent in time series data. These methods have demonstrated efficacy in predicting price movements across a wide array of commodities, providing valuable insights for traders, investors, and policymakers alike.
However, the advent of Artificial Neural Network (ANN) architectures has ushered in a new era of predictive modelling, offering unparalleled capabilities in capturing intricate patterns and nonlinear relationships within data. Architectures such as Long Short-Term Memory (LSTM) networks and Feed Forward Neural Networks (FFNNs), have emerged as powerful tools for forecasting grain yield and prices. (Liakos et al., 2018)
However, recognizing that no model can guarantee perfect predictions, traders often employ a combination of methods to enhance forecast reliability. Fundamental analysis, which examines supply and demand factors, economic indicators, and other tangible data, complements these econometric models. By integrating various methodologies, traders can form a more holistic view of the market, preparing for a range of potential outcomes and mitigating the inherent risks of relying on a single forecasting approach.
Conclusion
The strategic analysis of historical cash prices and freight rates is indispensable in the quest to forecast future movements in commodity markets. This comprehensive approach not only facilitates a deeper understanding of market dynamics but also equips traders and investors with the insights needed to navigate market volatility successfully and seize emerging opportunities. As commodity markets continue to evolve, the thoughtful use of historical data will remain a cornerstone of adjusting trading strategies, highlighting its value in an ever-dynamic economic and geopolitical landscape.
The meticulous analysis of historical data is not just a technical exercise but a strategic imperative that leverages patterns of the past, providing a foundation upon which future decisions of commodity trading strategies are made.
References:
Barkoulas, J., Hu, A. & Santos, M.R., 2008. The Link between Commodity Prices and Commodity-Linked-Equity Values during a Geopolitical Event. Academy of Accounting and Financial Studies Journal, 12, p.1.
Pai, P.-F., Hong, L.-C. & Lin, K.-P., 2018. Using Internet Search Trends and Historical Trading Data for Predicting Stock Markets by the Least Squares Support Vector Regression Model. Computational Intelligence and Neuroscience, 2018, p.6305246.
Bouoiyour, J., Selmi, R., Hammoudeh, S. & Wohar, M., 2019. What are the categories of geopolitical risks that could drive oil prices higher? Acts or threats? Energy economics, Vol.84, pp.1-14 https://researchdiscovery.drexel.edu/esploro/outputs/journalArticle/What-are-the-categories-of-geopolitical/
Carter, C., Rausser, G. & Smith, A., 2011. Commodity Booms and Busts. Annual Review of Resource Economics, 3, pp.87-118.
Liakos, K.G.; Busato, P.; Moshou, D.; Pearson, S.; Bochtis, 2018 D. Machine Learning in Agriculture A Review. Sensors 2018, 18, 2674. https://www.mdpi.com/1424-8220/18/8/2674
Recent Articles
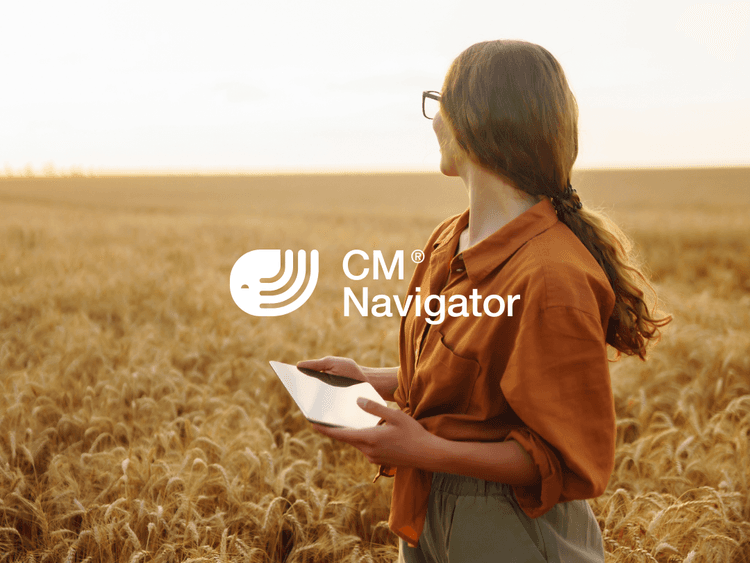
Article
Historical Data in Commodity Markets
Mar 15, 2024
In the complex world of commodity trading, the saying "history repeats itself" serves not merely as a philosophical thinking but as a foundational principle that supports investment and trading strategies. This is particularly relevant in...
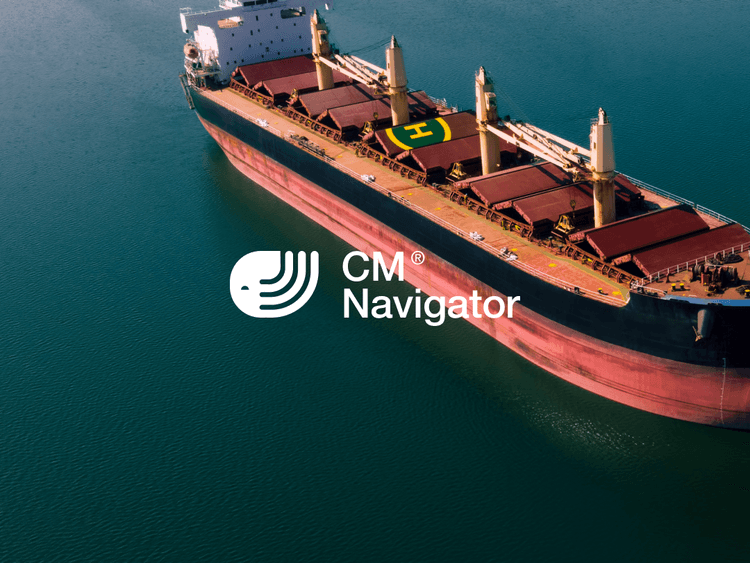
Article
Increased Transparency in Dry Bulk Freight Markets
Feb 05, 2024
Shipping markets have traditionally been opaque and closed, controlled by relatively few market participants placed in locations far away from demand for freight services, e.g. Greece, Monaco or Denmark. Given that ship supply in ...
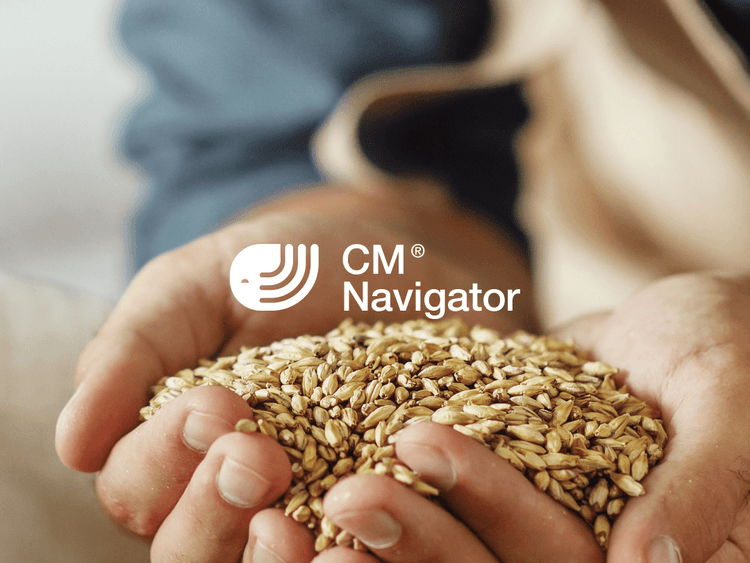
Article
Agricultural Supply and Demand Forecasting
Jan 02, 2024
In the global agricultural commodity markets, the ongoing ability to follow and forecast crop progress with accuracy is not just an advantage; it's a necessity to be able to compete. The interplay of multiple factors makes the ‘supply and demand dynamics’ complex...